[fit@hcmus TalkSeries 2019]
Chủ đề tiếp nối trong chuỗi hoạt động #TalkSeries2019 của fit@hcmus đó là:
1. Topic 1: Collective Online Learning of Gaussian Processes in Massive Multi-Agent Systems
Speaker: Dr. Hoang Trong Nghia
Ph.D. in Computer Science
The MIT-IBM Watson AI Lab of IBM Research
2. Topic 2: Budgeted Decision Making in Human-Aware AI Systems.
Speaker: Dr. Long Tran-Thanh, Assistant Professor in Computer Science - University of Southampton, UK.
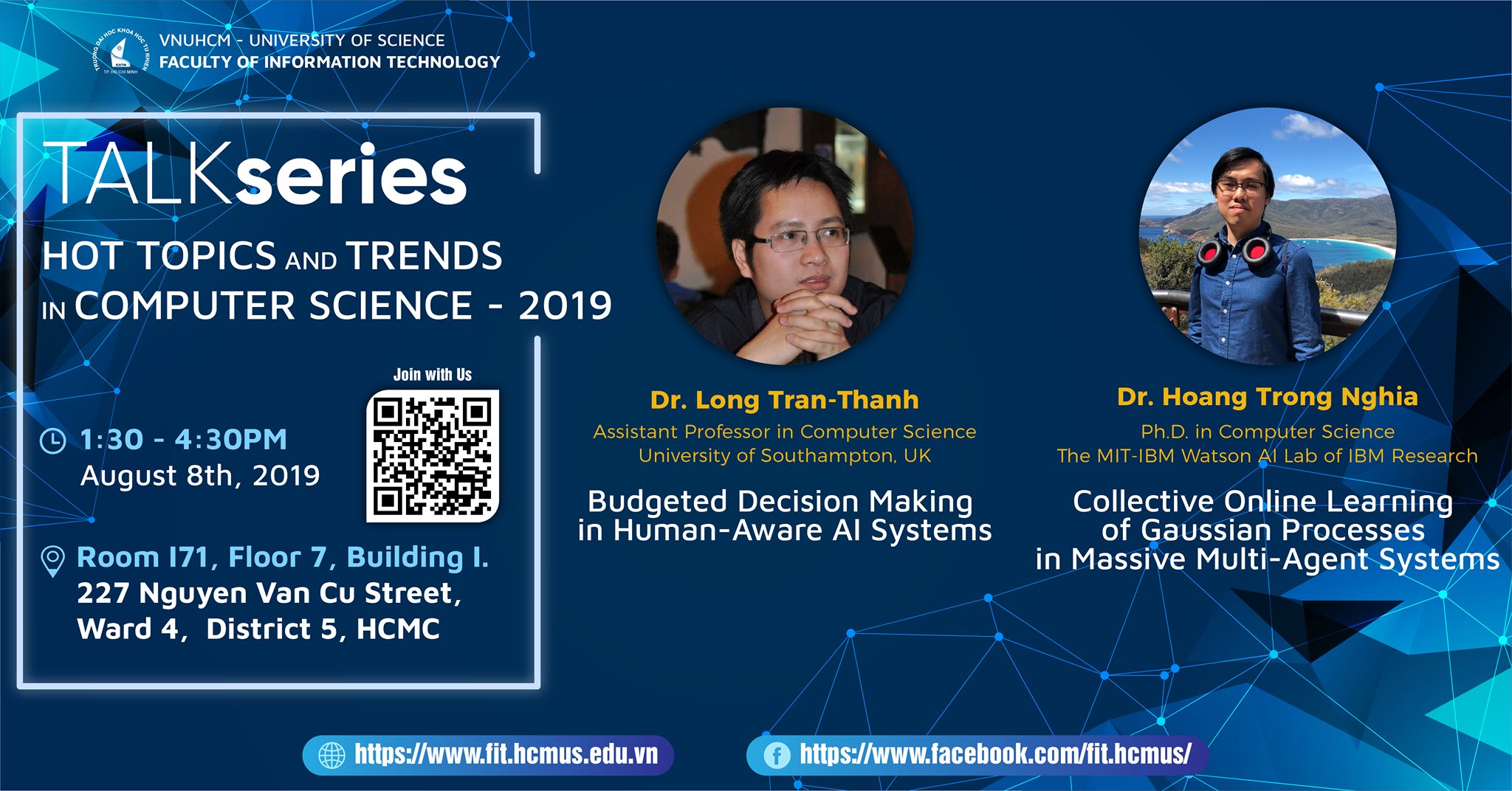
⏰Thời gian: 13:30 - 16:30, thứ 5 ngày 08/08/2019
����Địa điểm: Phòng I71, lầu 7, tòa nhà I. 227 Nguyễn Văn Cừ, Phường 4, Quận 5, TP.HCM.
���� Link đăng ký tham dự: https://forms.gle/SuwkXZajYGLqRZvk7 hoặc bạn có thể dùng QR code trên poster.
Do BTC có thêm 1 chủ đề, nên quý Thầy Cô, các anh chị và các bạn có thể lựa chọn chủ để tham dự thích hợp hoặc tham dự cả hai chủ đề đều được ạ.
⚠Hạn chót nhận đăng ký tham dự: 15:00 thứ 4 ngày 07/08/2019
Cần hỗ trợ thông tin gì thêm, vui lòng comment dưới bài post hoặc qua email: ntmdung@fit.hcmus.edu.vn
Trân trọng kính mời!
#TalkSeries2019
-------------
Abstract topic: Collective Online Learning of Gaussian Processes in Massive Multi-Agent Systems
Distributed machine learning (ML) is a modern computation paradigm that divides its workload into independent tasks that can be simultaneously achieved by multiple
machines (i.e., agents) for better scalability. However, a typical distributed system is usually implemented with a central server that collects data statistics from
multiple independent machines operating on different subsets of data to build a global analytic model. This centralized communication architecture however exposes a single
choke point for operational failure and places severe bottlenecks on the server's communication and computational capacities as it has to process a growing volume of communication
from a crowd of learning agents. In this talk, I will introduce a novel collective learning framework for massive distributed systems that allow each agent to build its local
predictive model, which can be exchanged and combined efficiently with others via peer-to-peer communication to converge on a global model of higher quality. I will also present some
empirical results that consistently demonstrate the efficiency of the proposed framework on several datasets.
-------------
Abstract topic: Collective Online Learning of Gaussian Processes in Massive Multi-Agent Systems
Due to the recent development of novel technologies such as Internet of Things (IoT), autonomous machines (e.g., driverless cars, or UAVs) and crowdsourcing, human-aware AI systems (i.e., systems in which humans and machines (or agents) have to perform some degree of cooperation with each other in order to achieve certain goals) are becoming a reality. In many cases, the required objectives of these collectives can be achieved via making a sequence of decisions under uncertainty. However, standard solution techniques for these type of problems fail to efficiently tackle these objectives, as they typically ignore the budget constraints caused by the human factor. For example, algorithms that require a sequence of iterative optimisation steps (e.g., gradient descent) assume that the participating agent (or human) will follow the steps of the optimisation process, described by the algorithm. However, humans might get disengaged or unmotivated, and thus, might leave the process, or intentionally do not follow the instructions given by the algorithm. Another example comes from the potential miscommunication between humans and agents, typically caused by inefficient interaction interfaces. Such new constraints introduce new challenges in solving sequential decision making problems of human-aware AI systems. Whereas some solutions have been proposed to tackle specific sub-problems with restricted settings, no effort has been made to date to investigate the problems of user motivation from a more fundamental and generic perspective, which is essential in order to fully understand these problems, and thus, to provide more efficient solutions to tackle them. In this talk, I will discuss some research solutions within each abovementioned topic. In particular, I will mainly focus on tackling budgeted human factors in sequential decision making problems, a research area I have been working on in the last few years.