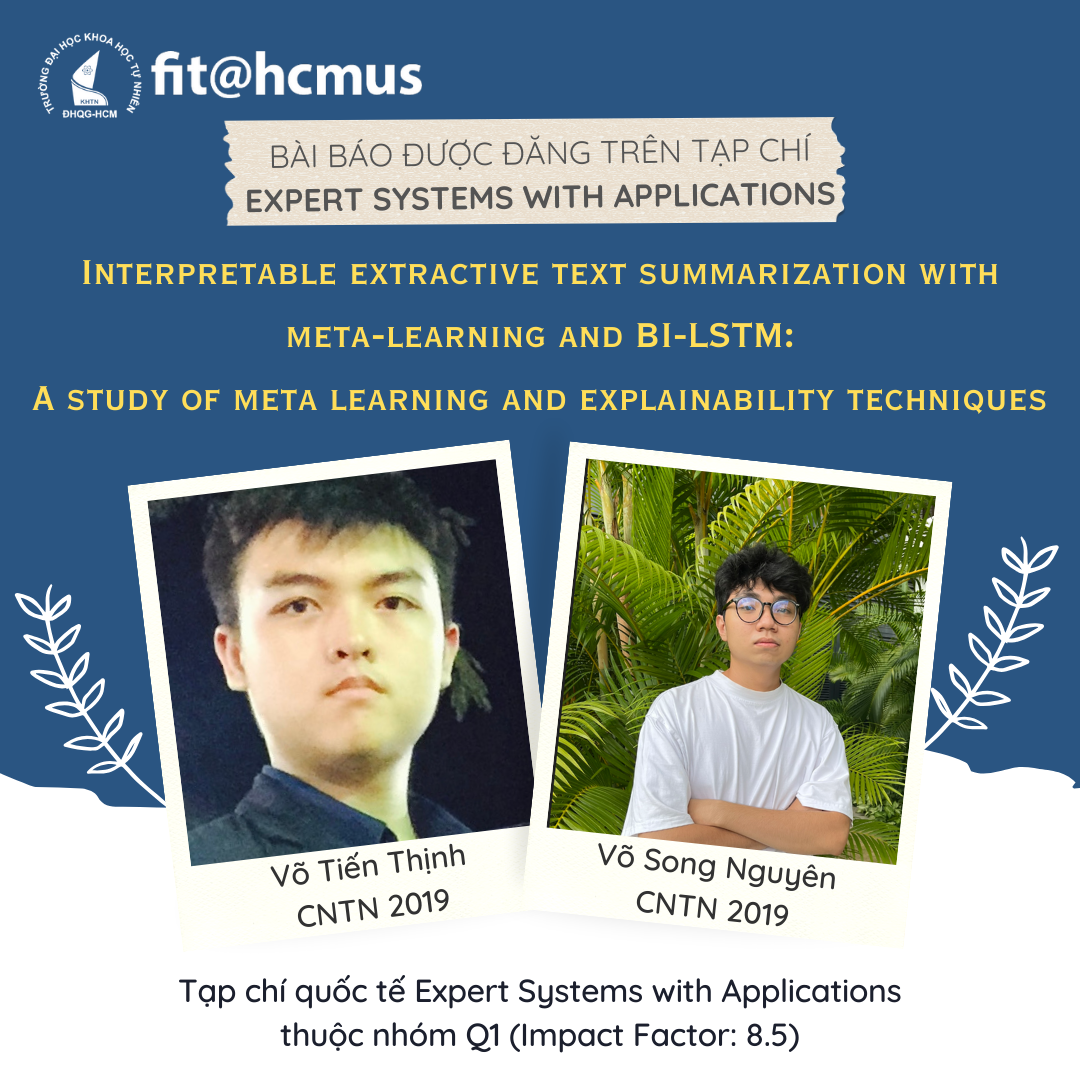
Nhóm sinh viên gồm hai tác giả chính Võ Song Nguyên và Võ Tiến Thịnh (cựu sinh viên lớp Cử Nhân Tài Năng khóa 2019, khoa Công nghệ thông tin) vừa công bố bài báo Interpretable extractive text summarization with meta-learning and BI-LSTM: A study of meta learning and explainability techniques trên tạp chí Expert Systems with Applications (SCIE-Q1, IF 8.5). Công trình của nhóm sinh viên này được thực hiện dưới sự hướng dẫn của GS.TS. Lê Hoài Bắc (Trưởng bộ môn Khoa học máy tính).
⭐️Điểm nổi bật của nghiên cứu: Giới thiệu phương pháp tiếp cận mới cho bài toán tóm tắt văn bản trong lĩnh vực xử lý ngôn ngữ tự nhiên (NLP), sử dụng meta-learning và BI-LSTM. Hiệu quả được so sánh với các mô hình NLP hàng đầu thông qua các chỉ số ROUGE.
Link bài báo: https://doi.org/10.1016/j.eswa.2023.123045
✅Tóm tắt bài báo: Text summarization is a widely-researched problem among scholars in the field of natural language processing. Multiple techniques have been proposed to help tackle this problem, yet some of these methodologies may still exhibit limitations such as the requirements for large training datasets, which might not always be possible, but more importantly, the lack of interpretability or transparency of the model. In this paper, we propose using meta-learning algorithm to train a deep learning model for extractive text summarization and then using various explanatory techniques such as SHAP, linear regression, decision trees, and input modification to gain insights into the model’s decision making process. To evaluate the effectiveness of our approach, we will compare it to other popular natural language processing models like BERT or XLNET using the ROUGE metrics. Overall, our proposed approach provides a promising solution to the limitations of existing methods and a framework for improving the explainability of deep learning models in other natural language processing tasks.